Fine-Grained Description of Image Semantics in Natural Language and Visual Question Answering
A quick glance at an image is sufficient for a human to point out and describe an immense amount of details about the visual scene. However, this remarkable ability has proven to be an elusive task for our visual recognition models.
Despite progress in perceptual tasks such as image classification, computers still perform poorly on cognitive tasks such as image description and question answering. Cognition is core to tasks that involve not just recognizing, but reasoning about our visual world. However, models used to tackle the rich content in images for cognitive tasks are still being trained using the same datasets designed for perceptual tasks. To achieve success at cognitive tasks, models need to understand the interactions and relationships between objects in an image. When asked “What vehicle is the person riding?”, computers will need to identify the objects in an image as well as the relationships riding(man, carriage) and pulling(horse, carriage) in order to answer correctly that “the person is riding a horse-drawn carriage”.
From Visual Genome Paper
Methdology
We developed methodology for automated image content description in natural language, using the combination of linguistic and visual information with the aim of better image understanding and visual recognition. Moving a step forward in this direction, Visual Question Answering (VQA) is a challenging task that has received increasing attention from both the computer vision and the natural language processing communities. Given an image and a question in natural language, it requires reasoning over visual elements of the image and general knowledge to infer the correct answer.
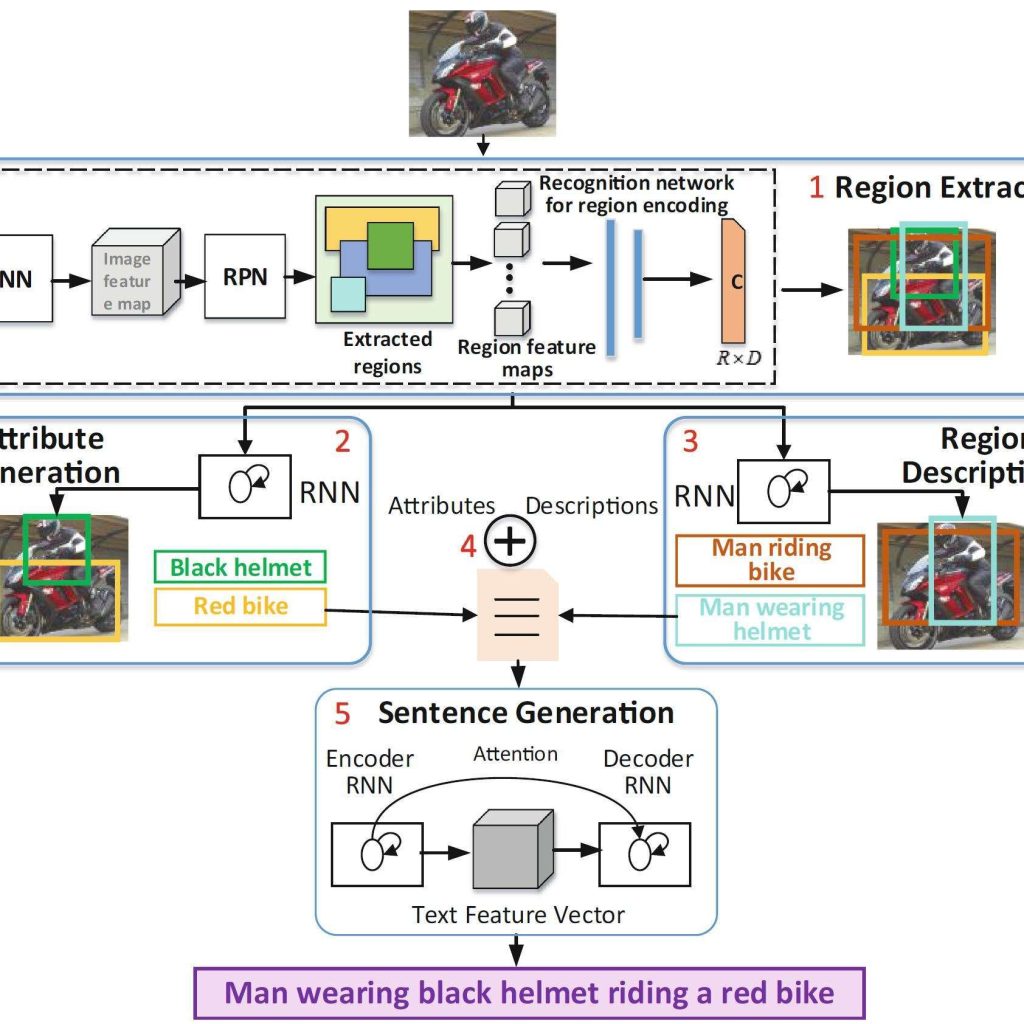
Faculty
Collaborator
Students
Selected Publications
- I Khurram, M.M. Fraz , M Shahzad, NM Rajpoot , “Dense-CaptionNet: A Sentence Generation Architecture for Fine-Grained Description of Image Semantics”, Cognitive Computing, Vol. 12 , No. 2, PP. 1-31, Mar, 2020. IF: 4.287
- I Khurram, M M Fraz, M Shahzad , , “Detailed Sentence Generation Architecture for Image Semantics Description”, Proceedings of the 13th International Symposium on Visual Computing, Nov, 2018, Las Vegas, United States.